SARS-CoV-2 has spread worldwide over the past years leading to a global pandemic. To date, this pandemic has infected over 590 million people and caused over 6.4 million deaths1. More severely, various persistent and prolonged sequelae are reported in convalescent patients, bringing more complex medical problems. Though vaccines are available, there inevitably shows intractable severe cases. Therefore, therapeutic antibodies are still needed for radical cure.
However, antibody design is a complicated protein design task, because SARS-CoV-2 families are famous for their highly variability. There are more than 10 recognized variants, in which alpha, beta, gamma, delta mutants have led global transmission and omicron is wreaking havoc now. The rapid mutations bring us a new challenge to conventional rational design – how to consider the effects of multiple amino acids on the binding sites simultaneously. Many of the designed antibodies have been proved to have reduced sensitivity or even lose their neutralizing activity when applied to new variants in the SARS-CoV-2 family, leaving an intractable problem in this field.
Protein-protein interaction is the basic mechanism for antibody-based therapy. Therefore, enhancing the specific affinity of antibodies becomes one of the most critical points of new drug development. With the traditional wet-lab based strategies, scientists introduce random mutations and further screening for antibodies with better functional property. However, such experimental approaches are very time-consuming and more importantly, cannot cover the large combinatorial space of amino acids. To address this problem, Helixon introduced a useful computational antibody optimization tool2, and has applied it on optimizing neutral antibodies against multiple severe acute respiratory syndrome coronavirus 2 (SARS-CoV-2) variants.
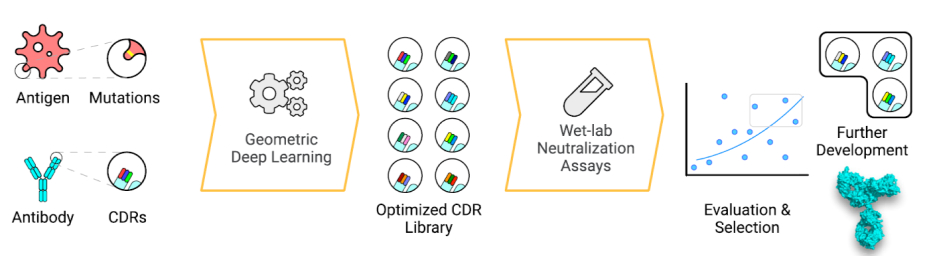
We trained a geometric deep learning model that could efficiently enhance antibody affinity to achieve broader and more potent neutralizing activity. Attention-based networks are capable of capturing the interaction features existing in the model. This property is perfectly utilized in our model to extract the inter-residue interaction features between the antigen and antibody.
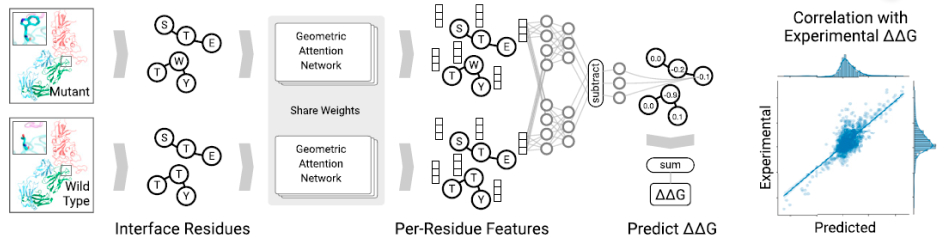
Based on this geometric embedding, the network learns to identify key residue pairs near the protein interface contributing to binding affinity, thus allowing us to measure the effects of mutation – the free energy change (ΔΔG). To search for favorable complementarity-determining regions (CDR), we simulated an in silico ensemble of predicted complex structures with CDR. The ΔΔG is set as the objective function in the deep neural network, helping to search for the best candidate.
We demonstrated the utility of our model on a human neutralizing antibody P36-5D2, which has strong potency against SARS-CoV-2 Alpha, Beta and Gamma, but not Delta variant. Our model shows great performance on optimizing this neutralizing antibody. In experimental validation, the predicted best CDR sequence did help the optimized antibody to improve the binding affinity against the Delta variant while maintaining activity against Alpha, Beta and Gamma. Through an iterative process of modeling and experimental validation, we were able to obtain six optimized antibodies with substantially improved potency of about 10- to 600-fold against multiple variants, also providing initial promising studies on Omicron.
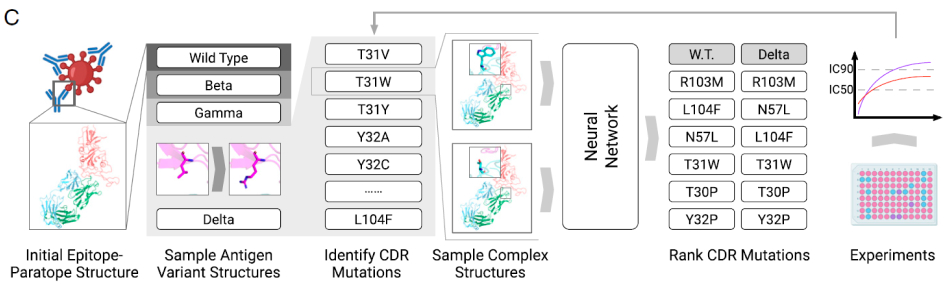
This AI solution for antibody optimization extends the limitation of traditional experimental methods in various aspects. Compared to random mutagenesis, computational simulation of CDRs enlarges the candidate pool to a great extent. More importantly, experimental mutations sometimes take away the critical functional residue of an antibody while they bring potential improvements. Computational strategy can avoid this problem and hence seek for optimal candidates.
The great performance and the outstanding properties of our model manifest the power of deep learning neural networks in antibody optimization and its potential application to engineering other protein molecules. This computational approach will not only bring more protein candidates that could be developed into promising antibody drugs, but also open the vision of artificially protein design.
“The new work marks what could be a milestone in AI: extending conventional wet-laboratory methods in infectious disease treatment by refining a traditional biological product with novel computer-driven methods.” – wrote in the report by ZDNet3
The code of ΔΔG prediction has been released on Github: Thttps://github.com/HeliXonProtein/binding-ddg-predictor